The Signal and the Noise – why local weather forecasters get it wrong, and what it means for those big market calls
I’ve finally got round to reading Nate Silver’s The Signal and the Noise. It’s a brilliant analysis of why forecasts are often so poor, from the man who called every state correctly in the 2012 US presidential election. In short, predictions are often poor because they are too precise (asserting an absolute outcome rather than assigning probabilities to outcomes); there’s often a bias to overweight qualitative information, gut feel and anecdote over data (these shouldn’t be ignored but must have a high hurdle to overrule the statistics); and there’s also a bias to ignore out of sample data (he suggests that the rating agencies mis-rated CDOs based on MBS because they assumed no correlation between housing defaults, which was indeed the case in the 25 or so years of US data that was used in the models. Japan’s property crisis statistics would have shown that in a downturn the degree of correlation in defaults becomes extremely high). I’d like to propose a deal though – we Brits agree never to use cricket statistics in any academic paper so long as Americans shut up about baseball. What the hell is hitting .300? How many rounders is that?
I liked these charts. The first shows just how good weather forecasting is nowadays. We can’t get the outcome right every time, but we can now call the probability of a weather event occurring right with the same probability of it happening. For example, when the US National Weather Service says that there is a 70% chance of rain, it rains 70% of the time. It snows 20% of the time when they say there is a 20% chance of snow.
But when your local TV weatherman gets hold of this same information, he or she distorted that information such that the outcomes were far worse than those of the National Weather Service’s forecasts. The chart below shows that local TV weatherpeople over-predicted weather events consistently. For example, if they say that there is a 100% chance of rain, it rains just 67% of the time, compared with the National Weather Service which if it says there is 100% chance of rain, it always rains.
Why? “Presentation takes precedence over accuracy”. In other words local TV news and weather people believe themselves to be entertainers as much as bearers of information. A firm prediction of a biblical rainstorm is more exciting that a range of probable outcomes, and a forecast for a scorching beach day more fun than assigning a 75% chance of sunny intervals. In other studies it was shown that political analysts on panel shows performed extremely badly, systematically predicting outcomes way out of line with statistical polling. The very act of being on TV reduces one’s forecasting ability. I think there is a likelihood that this is also true of economic and market forecasting, which is why market TV channels are full of people either calling for the Dow to soar another 200%, or for the global economy to collapse into a permanent ebola fuelled zombie apocalypse. There’s a danger that when we get phoned by journalists for comment we feel the need to be significantly away from the consensus, on payrolls, on the year end 10 year Treasury yield, on the chances of the Eurozone breaking up – and I’m sure I’ve been guilty of this too in the past. What’s more I’m sure that those who forecast extreme events end up being boxed into a corner where they feel they have to implement those views within portfolios, and end up with portfolios which point only in the direction of tail events and can’t perform in normal economic circumstances. I think this is a must read book for economists and fund managers to help us understand how good forecasts are made, and that the “loudest” forecasts get disproportionate airtime – and are often wrong. Silver has bowled a wicket maiden with this one.
The value of investments will fluctuate, which will cause prices to fall as well as rise and you may not get back the original amount you invested. Past performance is not a guide to future performance.
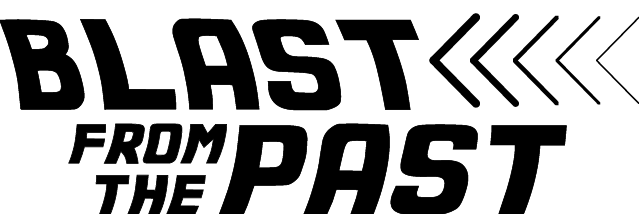
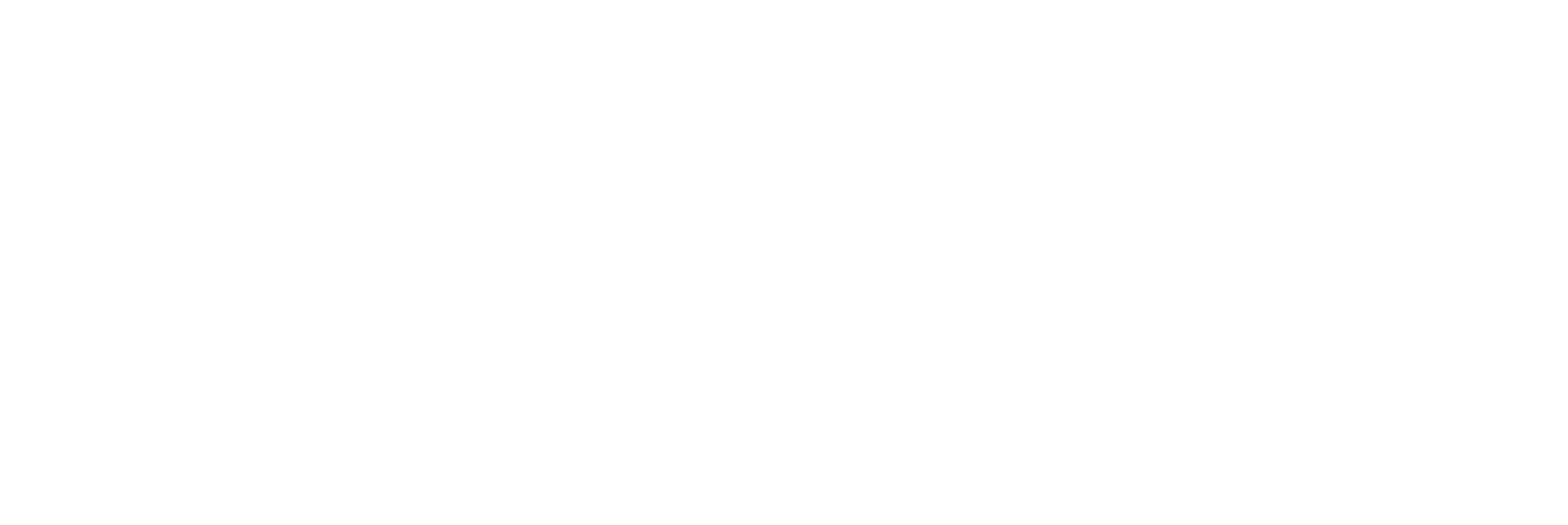
18 years of comment
Discover historical blogs from our extensive archive with our Blast from the past feature. View the most popular blogs posted this month - 5, 10 or 15 years ago!
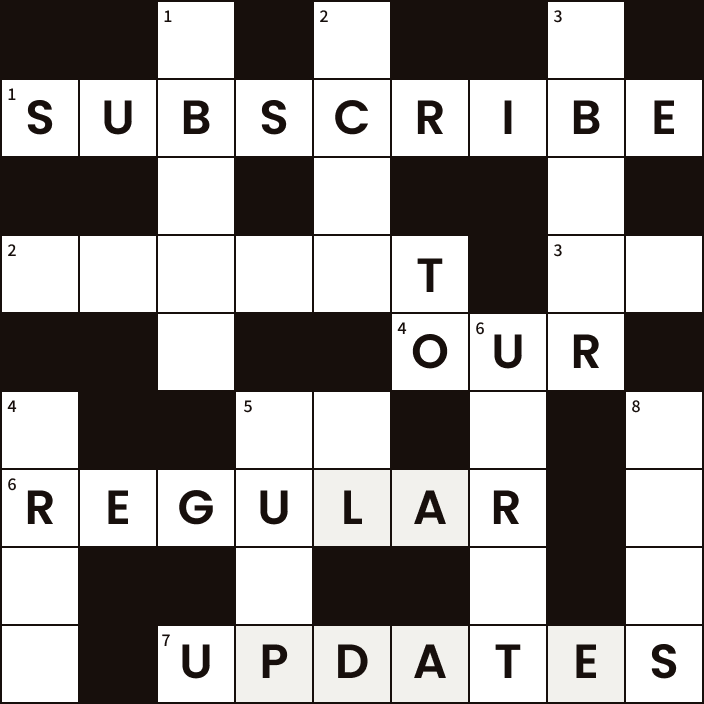
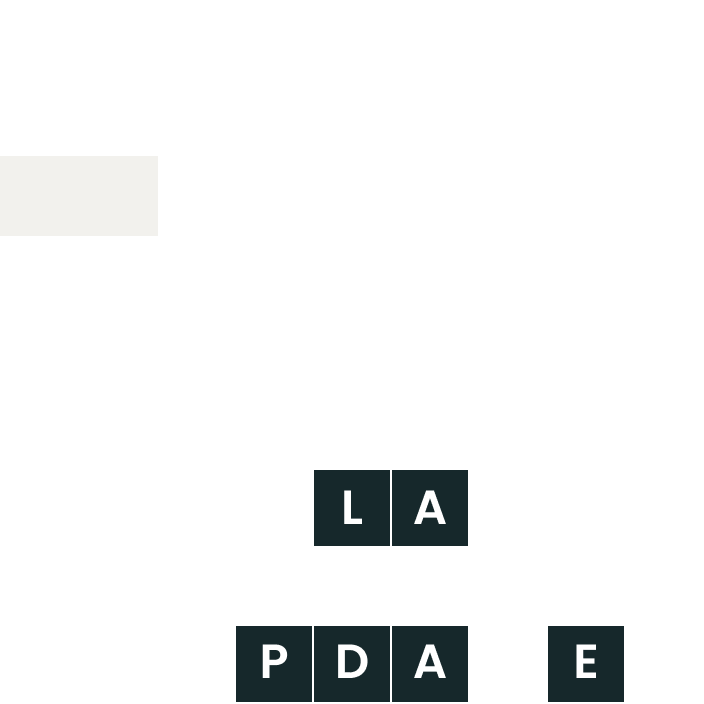
Bond Vigilantes
Get Bond Vigilantes updates straight to your inbox